The Rise of AI Voices: A Deep Dive into Neural Text to Speech
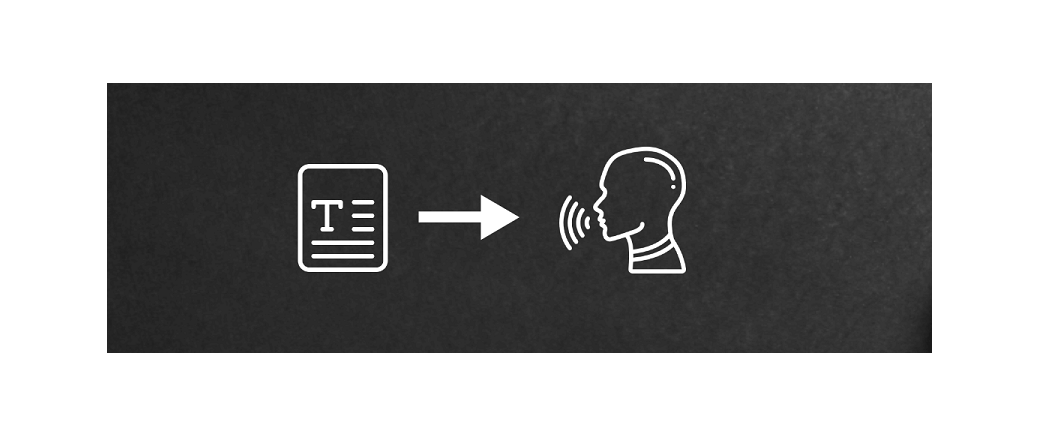
Imagine being able to translate text into spoken words in a manner that truly mimics human speech. Once a far-fetched idea, this has now become a reality with the advent of neural text to speech (NTTS) technology. NTTS represents a significant leap forward in the realm of speech synthesis, enabling us to generate human-like speech from written content with unprecedented accuracy and naturalness. In this blog, we will delve into the intricacies of NTTS, exploring how it works, its applications, and what the future holds for this exciting technology.

Table of Contents
What is Neural TTS?
NTTS is a type of speech synthesis that uses artificial neural networks to generate natural-sounding speech from text. It involves training a neural network, which is a computer architecture modeled on the human brain, on large amounts of speech data and then using the network to generate audio by converting texts into a sequence of acoustic features. The resulting speech can be highly expressive and used in a wide range of applications, including virtual assistants, audiobooks, and language learning tools, among others.
For a long time, TTS systems were known to generate robotic and monotonous-sounding speech, but recent advances in neural voices have led to significant improvements in the quality and naturalness of synthetic speech. NTTS systems have revolutionized voice synthesis with the power to generate realistic-sounding, high-quality audio with proper prosody, pitch, rhythm, and intonation.
How Does Neural Text to Speech Differ From Traditional Text to Speech?
Traditional text to speech systems use rule-based or statistical models and techniques to synthesize speech from text. These systems typically rely on pre-defined linguistic and acoustic models to generate speech. As such, the output lacks natural prosody, rhythm, and intonation. In contrast, NTTS software is trained end-to-end on large amounts of speech data, allowing them to learn the complex relationships between text and speech. As a result, NTTS systems can generate high-quality speech with natural prosody that closely resembles the human voice. Let's dive deeper to understand the differences between the two.
Prosody Transfer
NTTS systems can transfer prosodic features, such as stress, emphasis, intonation, and rhythm, from one voice to another, which allows for more control and customization of the generated speech to get the desired output. This is particularly useful for voice-based applications, such as voice assistants, where users may prefer a specific voice or speaking style. NTTS systems use a single end-to-end neural network to simultaneously perform both prosody prediction and voice synthesis. This integration results in more natural and human-like speech.
Traditional TTS, on the other hand, divides the process of generating speech into separate parts, with different models responsible for linguistic analysis and acoustic prediction, which often leads to inconsistent or unnatural prosody in the generated speech.
Speaker Adapted Models
Neural TTS models use deep neural networks to learn the relationship between text and speech from data, including the specific characteristics of a speaker's voice. Hence, it can be adapted to produce speech in the voice of a particular speaker with only a small amount of training data. Traditional TTS systems, however, require significant manual effort to create voices for specific speakers.
Emotional Speaking Styles
Emotional speaking styles add expressiveness and believability to synthesized voices. Unlike traditional TTS systems, which often struggle to produce emotionally expressive speech and fail to express emotion unless trained with huge loads of data, NTTS models can be trained to produce audio in different emotional tones, such as happy, sad, or angry. This makes the AI speaker more efficient and adaptable to different contexts and applications.
Evolution of Neural Text to Speech
In its early days, TTS systems were limited in their ability to produce expressive and emotionally-rich speech. To generate realistic voices, TTS systems needed to model the complex dynamics of the human vocal system. However, with the development of deep neural networks and large-scale speech datasets, NTTS systems have greatly improved their ability to produce more realistic speech.
Deep learning has enabled NTTS systems to learn the complex patterns of human speech from scratch and replicate it. These systems incorporate emotion-specific acoustic features into the neural network, allowing it to modify the tone and pitch of generated voices to convey different emotions.
Furthermore, early NTTS models required large amounts of data to train effectively, but newer models have been developed that require fewer data. This has made it easier to develop new TTS systems tailored to specific languages or dialects.
Advantages of Neural Text to Speech
Neural TTS systems offer several benefits, some of which are listed below.
Reduced Fatigue: Implementing neural voices in AI-based IVR systems has improved the user experience by reducing fatigue when interacting with the system. NTTS has led to a more genuine and fluent flow of conversation, which makes it easier for users to understand and engage with the chatbot. It has also made interactions more seamless and less frustrating, as the chatbot is able to understand the user's requests better and respond more realistically.
Natural and Engaging Interactions with Chatbots: NTTS has also made interactions with chatbots more natural and engaging. This is because the technology allows access to natural-sounding voices, which makes it easier for users to understand and engage with the AI speaker. Using neural voices in chatbots has resulted in positive experiences for users.
Emotion in Voices: One of the key benefits of neural voices is their ability to deliver emotions like happiness, sadness, and anger to voices. This has resulted in creating enhanced emotional engagement and user experience, particularly for applications such as virtual assistants, conversational agents, and customer support systems.
TTS Software That Use Neural Text to Speech
Today, there are several TTS software in the market that leverage NTTS techniques at their core to create and deliver a more realistic audio experience, including:
Natural Readers
WellSaid Labs
Amazon Polly Text to Speech
TTS Reader
FakeYou
Speechify
Why is Murf the Best Neural Text to Speech Software?
There are several factors, such as the naturalness and expressiveness of the neural AI voices, the range, and customization options that offer Murf the edge over other neural TTS software.
Language Options and Natural-Sounding Voices
Having a neural TTS tool with versatile language options is important for users to be able to reach a wider audience. With multiple dialect options available, users can communicate with a broader range of people and increase the impact of their content. That's why you need Murf, which has 120+ realistic voices in 20+ languages. With Murf, you can target both Chinese and Romanian audiences.
Voice Manipulation
Neural voices sound realistic but may not always be enough to achieve the desired output. This is where voice customizations play their part in bringing a more human touch to the output. Voice customizations can help create a voice that accurately reflects the user's desired tone, making the content more engaging and impactful.
Murf offers several voice customizations, including speed, pitch, pronunciation, emphasis, and volume. By manipulating these variables, users can create a unique voice that stands out and captures the attention of their target audience.
Voice Cloning
Another unique feature offered by Murf is voice cloning, with which users can create a clone of their desired voice and use it across different content. This feature is particularly useful for brands and content creators who want to improve their brand presence through voice.
Voice Changer
Murf's voice changer enables you to modify the gender of a voice in any existing voiceover or enhance the quality of a home-recorded speech to a professional studio-quality voiceover narration.
API
With Murf's API, you can integrate Murf's versatile voice generation capabilities into your products, applications, and workflows to unlock new features for your users.
In Summary
With its ability to synthesize realistic and expressive speech from texts, neural TTS is already being used in various applications to provide a more engaging and accessible customer experience. Looking ahead, the future of neural TTS is bright as researchers and developers continue to push the boundaries of what is possible with this technology. Some potential areas of development include:
Enhancing the robustness and adaptability of neural TTS systems, enabling them to handle various linguistic and contextual factors, such as accents, intonation, and background noise.
Integrating neural TTS with other artificial intelligence and machine learning technologies, such as natural language processing and computer vision, to create more intelligent and interactive systems that can better understand and respond to human needs and intentions.
Expanding the availability and accessibility of neural TTS, particularly for low-resource dialects and populations, through the development of more efficient and scalable training and deployment methods.
As the field continues to evolve, there are endless possibilities for further development and innovation!

FAQs
How does neural text to speech differ from traditional text to speech?
Neural text-to-speech denotes an important evolution from traditional text-to-speech systems. While the traditional approach mainly depends on rule-based or statistical models, neural text to speech leverages advanced neural networks trained end-to-end on broad speech data. Unlike old systems, neural text to speech does not depend on pre-defined linguistic and acoustic models; instead, it learns intricate text to speech relationships, captures nuances in pronunciation, intonation, and natural cadence directly from the data, resulting in remarkably human-like speech synthesis.
Can neural TTS handle multiple languages?
Yes, neural text-to-speech is perfectly designed to handle multiple languages. Having a neural text to speech with versatile language options is essential for users to reach a wider audience. This extensive language support empowers users to communicate with audiences from different linguistic backgrounds, including Chinese and Romanian. It ensures impactful communication, making it an indispensable tool for those seeking a global reach.
What is the difference between standard TTS and neural TTS?
The key difference between standard text to speech and neural text to speech lies in the underlying technology and the mode they generate speech in. Standard text to speech systems often use rule-based or statistical models with intricate dynamics of the human vocal system to synthesize speech from text. In contrast, neural text to speech has revolutionized voice synthesis with advanced neural networks and AI techniques. Unlike standard text to speech, it excels in incorporating emotion-centric acoustic features, producing human-like speech.
What applications can benefit from neural text to speech?
A notable advantage of neural voice text to speech is its ability to convey emotions like anger, sadness, and happiness, thereby enriching the user experience. This feature proves beneficial for various applications such as conversational agents, virtual assistants, audiobooks, language learning tools, and customer support systems, among others. The infusion of emotional touch elevates user communications, fostering an engaging communication experience.
Can neural TTS be applied to generate voices for virtual characters or avatars?
Yes, neural text to speech online can be used to generate voices for virtual characters or avatars. The innovative capabilities of neural text to speech lets you create natural-sounding and sensitive voices, which is vital for bringing virtual characters to life. The capacity to convey emotions in speech adds a layer of authenticity to virtual communications, making the characters more genuine. Whether in gaming or virtual simulations, employing this tool improves the overall user experience.
How does neural TTS enhance the naturalness of speech?
Neural voice text to speech systems excel in producing realistic-sounding, high-quality speech with proper tone, prosody, rhythm, stress, and intonation. By combining contextual awareness, prosody modeling, and high-quality waveform generation, neural voice text to speech systems produce synthetic voices that exhibit a level of naturalness and expressiveness. Unlike traditional text to speech systems that rely on concatenative or formant synthesis methods, neural text to speech leverages neural networks, specifically deep learning architectures like WaveNet and Tacotron, to mimic the complexities of human speech patterns.
What types of businesses or industries commonly use Neural text to speech?
Neural text to speech is extensively employed across diverse industries for its versatile applications. Businesses in e-commerce leverage it for engaging virtual assistants, while the entertainment and gaming industries benefit from realistic character dialogues. Educational platforms enhance e-learning content with dynamic accessibility and narration services, making information more accessible. From content creation in media to healthcare applications, it has been proven invaluable in enhancing communication across varied industries.
How is the quality of neural text to speech online maintained across different languages?
The quality of neural text to speech online is effectively maintained across diverse languages by comprehensive training on multilingual datasets. This includes capturing nuances in pronunciation, intonation, stress patterns, and rhythm specific to diverse linguistic contexts. Additionally, fine-tuning the text to speech models through language-specific training iterations, deployment of techniques such as transfer learning and data augmentation to supplement training data, and continuous monitoring, evaluation, and refinement enhance the quality and consistency of text to speech output across various languages.
Can neural text to speech be customized for specific industries or domains?
Yes, neural text to speech can be seamlessly customized for particular industries or domains, offering a customized and human-like output. This includes adjusting tone and pitch tailored to specific languages or dialects. Customization ensures the speech aligns flawlessly with the context and requirements of varied industries, whether for virtual assistants, educational content, or any other specialized domain.
Can neural TTS be used for audiobook narration?
Yes, neural text to speech is an ideal solution for audiobook narration, providing an immersive experience. Many platforms provide access to various AI voices, allowing users to infuse emotions for a remarkable listening experience and incorporate different voices for different characters.