How to Create an AI Voice? Learn Simple and Advanced Methods

developer resources and content, join us on our free Discord community.
AI-driven voices are revolutionizing how we interact with technology, providing a more natural and human-like communication experience. They are essential because they mimic human speech, understand context, and interpret language. From automating customer service interactions to crafting captivating content in entertainment and enhancing educational platforms with virtual tutors, AI voices are emerging as vital tools.
As AI voice technology becomes increasingly crucial in fields like entertainment, customer service, and education, knowing how to create a synthetic voice can be a valuable skill.
Two primary methods for creating an AI voice are using AI generators or building a custom voiceover from scratch. Each approach has advantages and challenges, catering to different needs and expertise levels.
Let's explore how to make an AI voice using voice technology and advanced custom techniques, offering step-by-step guides for each method.
Method 1: Creating an AI Voice Using an AI Voice Generator
AI voice generators convert text into realistic speech in just a few steps. They can help users create custom voiceovers for videos, create their own AI voice, or create personalized virtual assistants, acting as a tool for streamlining businesses and content creation.
What Is an AI Voice Generator?

AI Voice Generators are innovative software applications that harness the power of artificial intelligence to transform text into speech that sounds remarkably natural.
These tools make it simple to create lifelike voices through text to speech (TTS), voice changers, and voice cloning. AI voice generators allow users to create natural sounding voices effortlessly; no advanced technical knowledge in machine learning or speech synthesis is needed. The ease and speed provided by AI generators stand out as one of their major benefits.
Why get bogged down in the intricate and lengthy task of creating an AI voice from the ground up? Users can easily input text and have a voice generated in just minutes! These are perfect for content creators, marketers, and businesses looking for voices for videos, podcasts, virtual assistants, or presentations.
A number of AI voice generators provide a variety of customizable voice options across multiple languages and accents. These tools find their place in everyday applications such as virtual assistants, e-learning platforms, audiobooks, and advertising, offering a swift and budget-friendly way to incorporate voice into projects without the need of advanced technical skills or expensive voice actors.
Individuals opt for a free AI voice generator as they provide a quicker, more convenient method to produce high-quality voices without the intensive resource requirements of developing models from the ground up.
Creating an AI Voice with Murf: A Step-by-Step Guide
Murf AI is a leading voice generator platform that offers a wide range of customizable voice options. This makes it ideal for creating high-quality voiceovers and audio content in minutes.
Here’s how to create artificial voices using Murf:
Step 1: Sign up and Log in to Murf AI
Visit the Murf AI website and create an account. You can sign up with an email address or click on Sign up with Google, Microsoft, and Slack for easy access.

Step 2: Create a New Project
After logging in, click on Create Project. Give a Project Title and you can also create a folder in which you can have multiple projects.
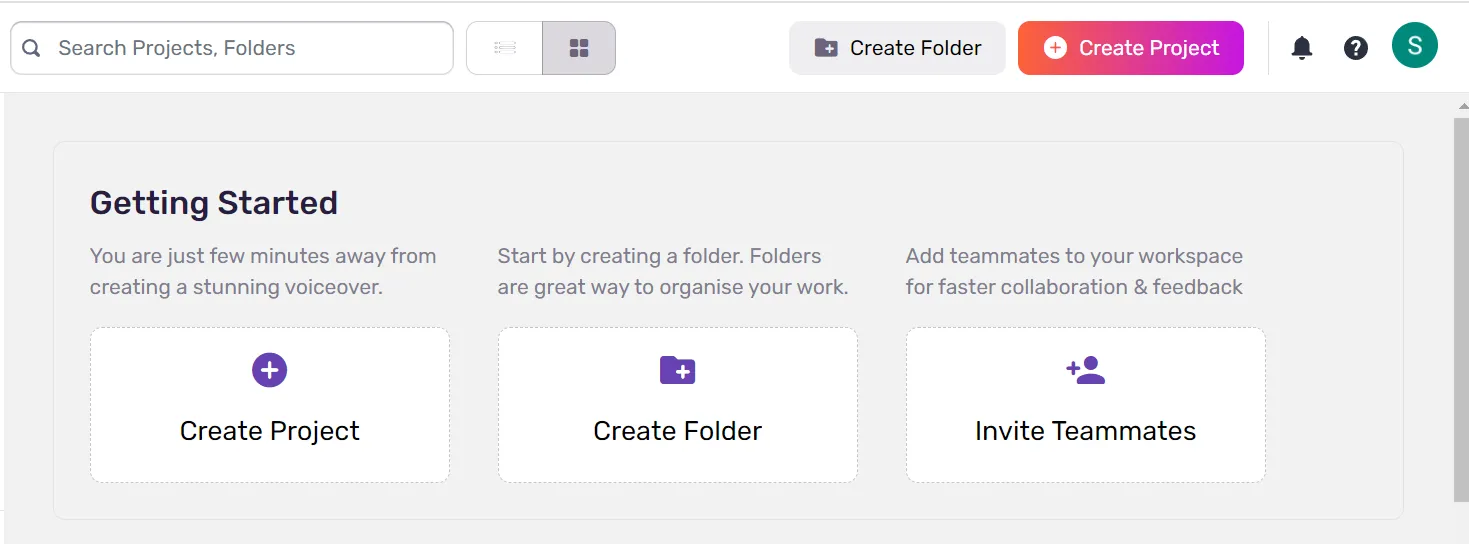
Step 3: Input the Text
Enter the text you want to convert into speech. Murf allows you to paste or upload written content directly into the text editor. You can divide the text as per line or paragraph or edit the entire text in a single block.

Step 4: Choose AI Voices
Murf offers 120+ voice options across 20+ multiple languages and accents like English, French, and Spanish. You can browse through available voices and listen to samples to find the one that best suits your project. Options range from friendly, conversational, and cheerful voices to storytelling, furious, and terrified tones.
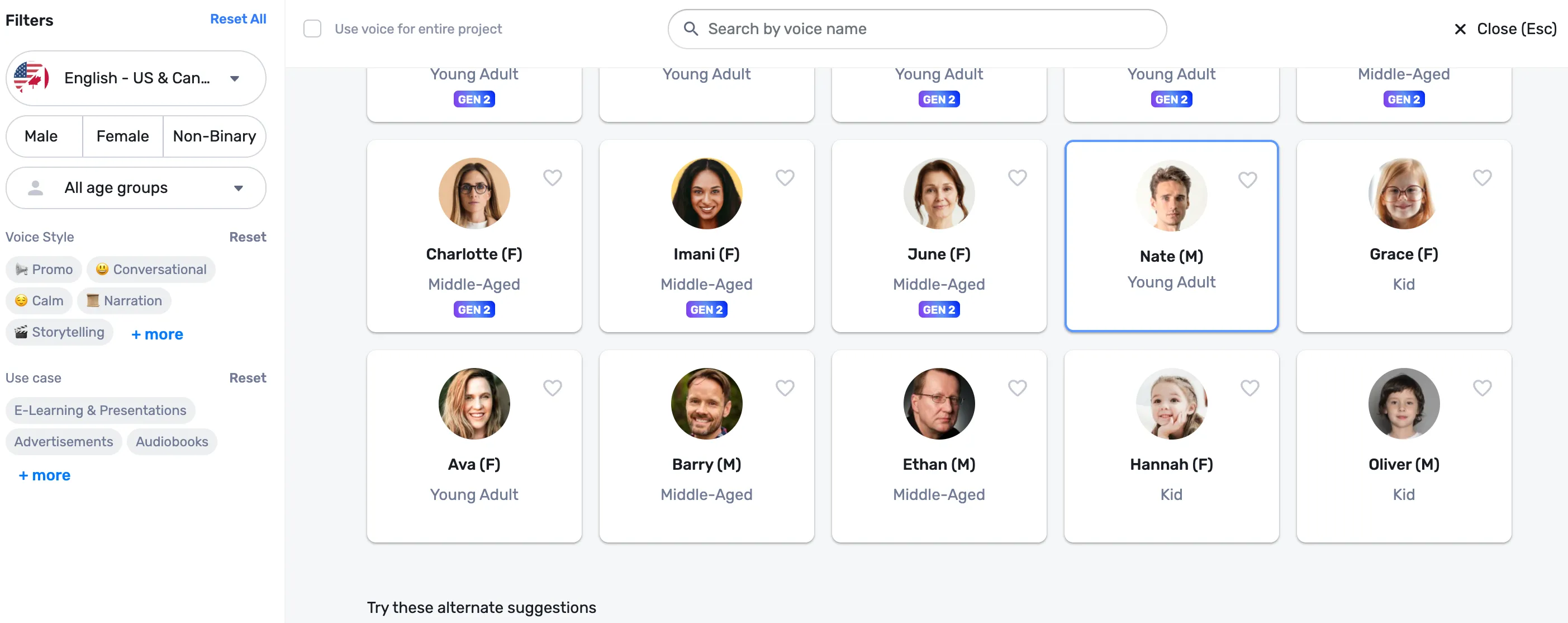
Step 5: Customize the Voice
Once you’ve chosen a voice, you can customize it by adjusting speed, pitch, emphasis, and pause settings. The "Say it My Way" feature allows you to record your own voice and direct the model to capture the intonation, tempo, and pitch of your produced speech.

Step 6: Preview and Fine-Tune
After customizing the voice settings, you can preview the output to ensure it sounds exactly as you want. You can also make adjustments to the written text or voice settings as necessary.

For example, suppose certain words need more emphasis or the pronunciation of a specific term needs adjustment. In that case, the word-level control feature allows you to tweak emphasis, pitch, or even the timing of individual words.
Step 7: Generate and Export Realistic AI Voices
Once satisfied with the final product, you can generate AI voices. Murf provides an option to download the audio files in various formats (MP3, WAV), making it ready for use in your projects.
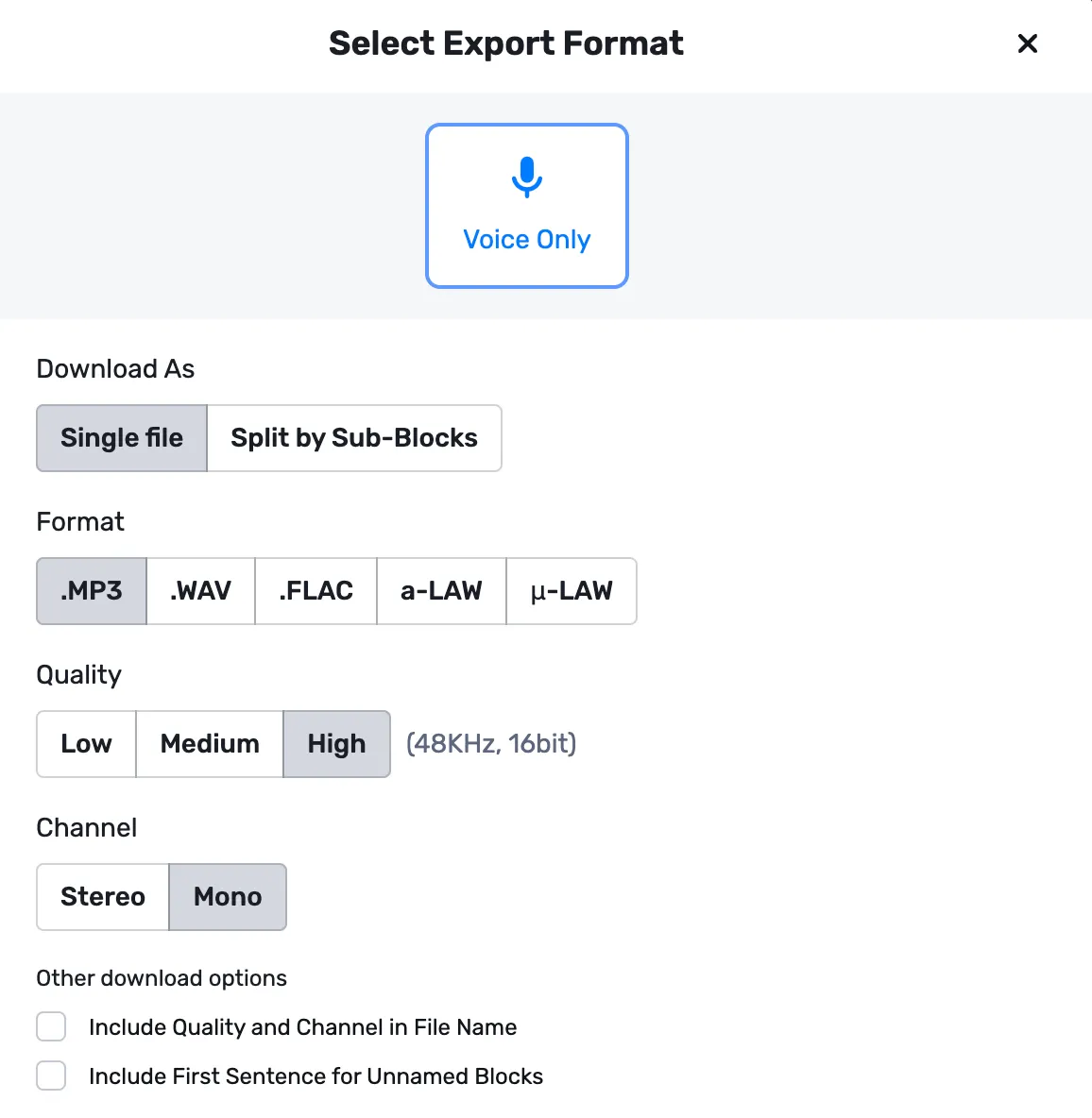
Read More: Explore Other Top AI Voice Generators of 2024
Method 2: Creating Natural sounding AI Voices from Scratch
Building an AI voice from scratch is the more technical approach for total control and customization of the output. This approach teaches AI models to synthesize human-like speech depending on data sources.
Although this approach requires more time and resources, it has the advantage of producing a highly personalized synthetic voice with exact control over its tone, style, and other subtleties.
How to Make an AI Voice: A Step-by-Step Process
Building an AI voice from scratch requires many stages, from data collection to model deployment.
Step 1: Collecting and Preparing Data
Getting high-quality speech data entails compiling voice samples as the basis for training the AI model. A strong dataset requires a lot of audio data with varied speech patterns, accents, and intonation. Once the data is acquired, it must be cleaned and labeled appropriately.
Step 2: Choosing a Speech Synthesis Model and Setting Up the Environment
Next, we select a speech synthesis model as the AI foundation for voice production. To operate with PyTorch or TensorFlow machine learning models, you must create the required development environment.
In this environment, you will build and train the model, requiring a high-performance configuration to analyze vast volumes of data.
Step 3: Training the Model with the Collected Data
The next step is to train the AI model with the gathered data. Training provides the model with text and voice data, allowing it to learn how to translate text into naturally occurring speech.
Training can take a significant period, depending on the dataset's size and the model's complexity. The model keeps improving at predicting how words should sound, their pronunciation, and the suitable emotional tone for various settings.
Step 4: Fine-Tuning the Model for Better Accuracy and Naturalness
After the first training, the model is tweaked to improve the speech quality produced. In addition to correcting any pronunciation errors, developers might adjust the tempo, pitch, and voice output clarity.
Step 5: Testing and Deploying the AI Voice
Lastly, the AI voice is tested and implemented. During this step, developers run the model through a set of test cases to ensure that the synthetic voice faithfully reproduces several kinds of input text naturally and consistently.
AI Voice Generator: Challenges and Considerations
Although building AI voices from scratch provides unmatched customization and control, there are also significant ethical questions and issues involved.
Common Challenges
Creating natural-sounding AI voices from scratch can introduce the following challenges:
1. High-Quality Data Requirement
One of the toughest obstacles in producing AI voices is obtaining high-quality speech data. The dataset must be varied and include a broad spectrum of speech patterns, emotions, and pronunciations.
Insufficient or low-quality data might result in poor voice output with pronunciation, intonation, and general clarity issues.
2. Significant Computational Resources
Teaching a machine-learning model to produce human-like speech requires significant computational capability. The heavy processing required calls for either cloud-based infrastructure or high-performance servers.
3. Expertise in Machine Learning
AI synthetic voices from scratch require an advanced understanding of speech synthesis technologies, Natural Language Processing (NLP), and machine learning. Developers must know how to gather and prepare data, train models, and adjust settings.
Ethical Considerations
AI voices also have the following ethical challenges to consider:
1. Potential Biases in AI Models
Should the AI speech model's training data include prejudices such as gender, ethnic, or cultural ones these will show in the voice output.
For instance, an AI voice may sound more natural when using a standard American accent but struggle with non-native accents or minority dialects, leading to mispronunciations or inauthentic speech patterns. This leads to an uneven user experience, where the AI might appear more accurate or expressive for some groups while sidelining others, which could reinforce stereotypes or overlook diverse voices.
2. Ethical Implications of Synthesized Voices
Impersonation and misleading behavior pose major risks, as realistic AI voices have the potential to generate deepfake audio files or replicate real people without authorization. Fraudulent activities, such as posing prominent personalities, celebrities, or even common people, can employ this technology to fool or control viewers.
To Wrap Up
AI generated voices are a great choice for individuals who need quick, dependable, and reasonably priced answers as they offer customization options and produce professional results in minutes.
Building AI-generated voices from scratch gives developers total control and customization, and it requires excellent data, computational tools, and machine learning knowledge.
AI Voice Generator vs. AI Voice from Scratch: The Better Choice
Choosing between using an AI voice generator or building a voice from scratch depends on your goals, time, and resources. For most users, leveraging a tool like Murf AI will be the best ai voice generator for it's practical and efficient route.
Ready to experience the power of AI voices? Try Murf AI today and transform your text into lifelike speech effortlessly.
Sign up below and see how Murf can elevate your projects with state-of-the-art voice technology!
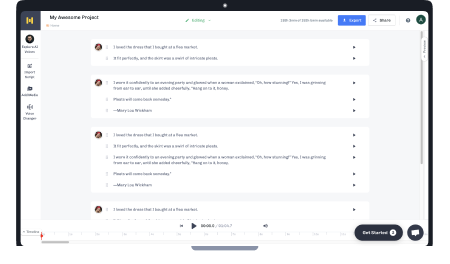
Frequently Asked Questions
What’s the difference between using an AI voice generator and creating an AI voice from scratch?
AI voice generators are simple tools that allow users to generate voices quickly, requiring no technical expertise. In contrast, creating an AI voice from scratch is a complex process that requires data collection, machine learning knowledge, and high-performance computational resources for total control over voice output.
How does an AI voice generator work?
AI voice generators use text to speech technology to convert written text into spoken words. These tools analyze the text input and create a human-like voice output by adjusting pitch, speed, and tone.
How long does it take to create an AI voice from scratch?
Building an AI voice from scratch is a time-consuming process. It involves collecting voice data, training the model, and fine-tuning the output, which can take weeks or even months, depending on the complexity and the amount of data.