The Evolution of Text to Speech Model

developer resources and content, join us on our free Discord community.
Text to Speech (TTS) has gained widespread use in consumer applications, including smartphones and home automation. It improves user experience by allowing devices to communicate with users through voice, making interactions feel more natural and easier to understand.
This voice-based interaction simplifies tasks like reading texts aloud and controlling smart home devices, making technology more accessible and convenient for everyone.
In fact, the global TTS market is expected to be valued at $4 billion in 2024 and grow to $7.6 billion by 2029, at a CAGR of 13.7%, with increasing demand from industries like automotive, healthcare, and education.
Advanced machine learning techniques and natural language processing play a crucial role in enhancing the quality of synthesized speech. These techniques are instrumental in training a text to speech model that lays the foundations for TTS technology.
Let's explore the various types of TTS models and the leading brands that are shaping the future of this technology.
What Are the Different Types of Text to Speech Models?
The different types of text to speech models that have evolved to meet diverse needs and applications:
1. Concatenative Synthesis Models
Concatenative Synthesis Models represent a traditional approach in the realm of text to speech (TTS) technology. These models function by using pre-recorded speech segments meticulously collected from actual human voices. Each segment, often a phoneme or syllable, is stored in a vast database. When a text input is received, the model selects appropriate segments and concatenates them to form coherent speech output.
This method ensures a high level of naturalness in the resulting speech, as the sounds originate from actual human recordings. For example, Murf AI uses this model effectively to provide clear and natural voice outputs for e-learning modules, carefully stitching together pre-recorded voice segments to ensure high audio quality.
Concatenative Synthesis is ideal for applications where clarity and naturalness are paramount, such as in automated customer service tools and navigational aids. Despite its benefits, this model requires significant storage for the speech database and can be limited in its flexibility compared to more advanced TTS models like neural networks.
2. Parametric Synthesis Models
Parametric Synthesis Models offer a versatile approach to text to speech (TTS) technology. They generate speech based on phonetic parameters rather than relying on pre-recorded voice segments. These models convert text into phonetic representations and then synthesize speech using parameters like pitch, duration, and intonation.
The model analyzes the input text and synthesizes the corresponding speech by adjusting these parameters to simulate natural speech patterns. The process occurs in real time, with speech being generated instantly as the text is processed.
This allows for faster response time and less memory usage since there's no need to store large audio files. While sometimes less natural-sounding than concatenative models, parametric synthesis offers various advantages in customizability and system footprint.
For instance, Parametric Synthesis is used in GPS systems because it can dynamically generate speech from text using predefined vocal parameters. Its ability to swiftly switch between languages and adjust parameters like pitch and intonation to mimic different accents or tones enhances the user experience.
This flexibility is effectively utilized in systems such as TomTom GPS, which relies on parametric synthesis for offering navigation instructions in multiple languages. It’s also used in multilingual tools like iSpeech, which offers dynamic voice responses in various languages, adapting to the user’s native dialect for more natural interaction.
3. Neural Network-Based Models
Neural Network-Based Models use statistical methods and machine learning to produce speech. They leverage extensive collections of speech samples to teach neural networks how to generate speech that imitates human intonations and rhythms.
Instead of using pre-recorded clips or synthetic voice sounds, these models examine language and speech patterns to produce more natural and smooth output.
For instance, Google's Tacotron model is a perfect fit for interactive voice response systems as it is trained on a large dataset to replicate human speech nuances. This feature enables the models to more accurately adjust to different speech styles, accents, and languages.
4. End-to-End Deep Learning Models
End-to-end deep Learning Models are at the forefront of TTS technology. They utilize advanced neural networks and deep learning to deliver highly realistic speech. By processing text directly to speech and bypassing traditional stages like phonetic processing, these models achieve remarkable efficiency.
Trained on extensive spoken language datasets, they develop nuanced vocal patterns that mimic human speech intonations and expressions. The end-to-end models are designed to improve over time, continuously learning from new interactions to enhance speech accuracy and naturalness.
Additionally, these models can struggle with extreme speech variations, like rare accents or dialects not covered in their training data. They may also respond inaccurately to unexpected phrases or idioms, resulting in less natural speech output.
A prime example is OpenAI’s Whisper model, which processes extensive linguistic data to tailor speech for various contexts and user interactions, thereby enhancing virtual assistant functionalities.
These models can transform the way individuals with visual impairments interact with digital content by providing more intuitive and responsive audio descriptions. Additionally, in educational settings, they can deliver tailored instructional content that adapts to the learner's pace and style.
Brands Leading in Text to Speech Technology
Several leading companies have developed their own text to speech (TTS) models, each utilizing different technologies to meet specific user needs.
Here’s a brief overview of the best text to speech models worth exploring in 2024:
Murf AI
Murf AI uses cutting-edge neural network-based TTS models, with its latest iteration, Gen2 represents a significant leap forward in voice quality and expressiveness. This second-generation model produces human-like speech, capturing intricate nuances and subtleties.
Operating at a 44.1 kHz sampling rate, Murf Gen2 spans the full human audible range, delivering exceptionally clear and natural outputs. The platform supports over 20 languages, including regional accents in English, Spanish, Hindi, French, German, and Portuguese, with an advanced linguistic layer to ensure precise pronunciation and accent accuracy, even for low-resource languages.
What sets Murf AI apart is its ability to seamlessly blend linguistic precision with advanced neural modeling, creating speech that feels authentic and engaging.
Google TTS
Google utilizes both Tacotron and WaveNet models to power its text to speech technology. Tacotron transforms written text into mel-spectrogram representations for speech synthesis, effectively capturing the nuances of human speech, such as intonation and rhythm.
WaveNet, a deep neural network, generates highly natural and expressive speech from these spectrograms. It uses a revolutionary approach to produce one audio sample at a time, which allows it to emulate the intricacies of the human voice with unprecedented accuracy.
Together, they create a seamless user experience, particularly in applications like Google Assistant and Maps, where clear, human-like speech is essential.
Amazon Polly
Amazon Polly offers two main TTS models: neural TTS for high-quality, lifelike speech with adaptive intonation and standard TTS for a more basic but cost-efficient solution.
Neural TTS uses deep learning to create highly realistic, fluid speech, which is ideal for customer engagement where voice quality is crucial. Standard TTS, using traditional synthesis techniques, stitches together pre-recorded speech units, resulting in a more robotic tone but at a lower cost. It's suitable for applications where voice fidelity is less critical, such as automated responses in IVR systems.
Microsoft Azure TTS
Microsoft Azure's Neural network-based technology leverages deep neural networks to convert text into lifelike spoken audio. It supports a wide range of languages and dialects, including English, Mandarin, Spanish, French, and Arabic. This capability is crucial for applications that require inclusivity and accessibility worldwide.
Azure's TTS offers customization options for specific scenarios, such as adjusting speech speed, pitch, and emotion, making it adaptable for various industries. For instance, in education, it can power interactive learning tools and audiobooks, while in healthcare, it can provide spoken reminders for medications and appointments.
It also enhances customer service by improving the naturalness of responses in AI-driven chatbots and virtual assistants. This versatility and adaptability make Azure's TTS a powerful tool for creating accessible and engaging user experiences in multiple languages.
IBM Watson Text to Speech
IBM Watson's TTS technology, built on neural network-based models, delivers clear and accurate speech, particularly suited for business applications like virtual assistants and automated customer service. Its advanced machine learning algorithms enhance the speech's adaptability to various contexts, ensuring consistency and accuracy even in specialized or technical domains.
For instance, it provides technical support in IT by explaining system updates and troubleshooting steps in industry-specific language. Additionally, legal professionals can clearly articulate complex legal documents, enhancing accessibility and efficiency. In financial services, Watson's TTS helps in reading out dense financial reports and banking terms, improving the user experience on financial platforms.
In the automotive sector, it provides drivers with technical details about vehicle maintenance and navigation through auditory feedback. These applications demonstrate Watson's capability to handle linguistic complexities across different fields, making information more accessible and interactions more intuitive.
OpenAI Whisper
Trained on 680,000 hours of diverse, multilingual data, Whisper is particularly adept at handling a variety of speech patterns, accents, background noises, and domain-specific language.
Whisper’s applications are vast, including enhancing accessibility by providing real-time captions for the deaf and hard-of-hearing community, automating transcription for media and legal sectors, and creating voice-enabled interfaces for apps and devices.
Additionally, its open-source nature encourages innovation, enabling developers to integrate voice-to-text capabilities into their applications or experiment with further advancements in speech recognition technology
Companies such as Snap, Quizlet, and Truvideo are utilizing OpenAI's Whisper API to leverage its speech recognition capabilities across various industries.
Future of Text to Speech Models
Ongoing advancements in artificial intelligence and machine learning are set to drive significant progress in the future of text to speech (TTS) technology. These are a few trends that influence this landscape:
Advancements in Neural Networks
- Enhanced naturalness: Future TTS models will incorporate improved deep learning architectures like convolutional neural networks (CNNs) and recurrent neural networks (RNNs), trained on extensive and varied speech datasets. This training enables the models to capture subtle differences in speech patterns and intonations, allowing for more natural and contextually aware voice output. By analyzing a wide range of linguistic features, these models will better mimic human speech nuances, adapting to different emotional and situational contexts.
- Advanced Algorithms: Improved algorithms, including advanced deep learning models and attention mechanisms, will enhance TTS systems' ability to grasp context. This will enable a more accurate adaptation of tone and style, tailoring speech outputs to match the significance of the text and the intended user engagement. Techniques like prosody prediction and self-supervised learning further refine the naturalness and responsiveness of speech.
- Personalization: Future TTS models, building on neural network technologies like OpenAI's GPT and Google's Tacotron, will enhance customization through user feedback and advanced algorithms. These models will allow direct adjustments to voice attributes such as pitch, speed, and timbre and will adapt to individual accents and speech nuances, personalizing the voice output more effectively.
Enhanced Personalization and Naturalness
- Voice Customization: Individuals can adjust voice characteristics such as pitch, speed, and timbre in great detail to generate distinctive vocal styles for various purposes.
- Advanced TTS systems: Using cutting-edge technologies like WaveNet and Tacotron, advanced TTS systems will be capable of displaying a broader range of emotions and intonations. These systems, based on deep neural networks, are designed to enhance the dynamic and responsive feel of synthesized speech, making it more lifelike and engaging.
- Adjusting to User Comments: TTS technology will enhance performance by integrating user feedback through adaptive algorithms. These systems learn from user interactions, adjusting speech attributes like pitch and speed based on preferences, ensuring the voice output evolves to better meet individual needs over time.
For example, TTS can be tailored for individual learning experiences in educational apps, where it adapts the narration speed and complexity based on the learner’s proficiency. In healthcare, personalized TTS can help deliver patient-specific medical information in multiple languages, enhancing understanding and compliance.
Moreover, in customer service, TTS can provide a more personalized interaction by recognizing customer emotions and adjusting responses accordingly. Overall, with the advancement of TTS models, these systems can simulate more dynamic, human-like conversations, catering to various industries by enhancing user interaction, personalization, and emotional intelligence in synthesized voices.
Wrapping Up
Today's TTS models, spanning from neural network-based solutions to advanced end-to-end deep learning systems, have transformed the landscape of voice synthesis, enabling lifelike, context-aware speech generation.
These advancements enhance user experiences across numerous domains, such as education, healthcare, and customer service, while also broadening digital content accessibility for people with disabilities.
Looking ahead, the future of TTS technology promises further innovation. Enhanced neural networks, coupled with more personalized voice features, will create more dynamic and emotionally intelligent interactions. Applications such as virtual assistants, audiobooks, and interactive learning tools will benefit from these developments, offering users a tailored experience that adapts to their preferences and emotional context.
Embracing these innovations, Murf AI continues to lead with cutting-edge TTS solutions, ensuring that every voice interaction is compelling and authentic. Whether you’re looking to elevate your content, streamline business communication, or create more engaging experiences, Murf AI offers the tools to bring your voice projects to life.
Sign up below and take your voice projects to the next level today!
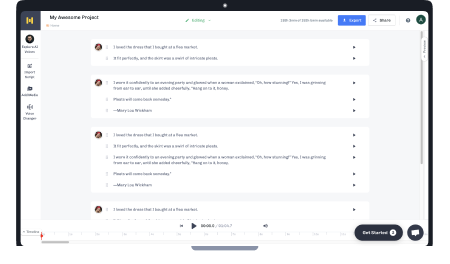
Frequently Asked Questions
What are text to speech models?
Text to speech (TTS) models convert written text into spoken words using computer algorithms. They are designed to enhance accessibility and improve user experience across various digital platforms by generating audible speech.
How do text to speech models work?
TTS models work by analyzing input text, breaking it down into smaller components like phonemes, and then generating speech based on those segments using either pre-recorded human voices or synthesized speech algorithms. Modern models, such as neural networks, are trained to produce highly natural and smooth-sounding speech.
Which TTS model is best for natural-sounding voices?
Neural Network-Based Models and End-to-End Deep Learning Models are considered the best for natural-sounding voices. They use advanced machine learning techniques to closely mimic human speech patterns, intonation, and expression, resulting in more realistic voice output.
How are TTS models trained and evaluated?
TTS models are trained using large datasets of human speech and text, allowing them to learn language patterns and speech characteristics. They are evaluated based on their ability to produce clear, natural, and contextually appropriate speech, often measured through user feedback and quality assessments like intelligibility and fluency tests.