TTS Model with 99.38% Pronunciation Accuracy
Text-to-speech technology has long struggled with accuracy and natural voice quality. Murf AI brings a new approach to how text becomes speech. We have trained our models on 70,000+ hours of professional voice recordings, and our deep linguistic analysis identifies how words should sound in different contexts and languages. This delivers high-quality speech and superior pronunciation accuracy that's true to life.
To establish this, we have conducted 3 tests that test the limits of our model and showcase its true capabilities in real-world scenarios.
1st Test - Pronunciation Accuracy
Study Overview
To evaluate Murf's pronunciation capabilities, we carried out blind tests focusing on real-world language usage patterns. These assessed how accurately our model pronounces words across multiple languages and common usage categories, providing a foundation for understanding our system's linguistic precision in everyday contexts.
Results & Analysis
The evaluation data shows that across all tested languages, Murf achieved pronunciation accuracy rates exceeding 98.7%. French achieved the highest accuracy at 99.75%, while UK English showed the most opportunity for improvement at 98.70%.
.webp)
These results indicate that the system effectively handles pronunciation across diverse linguistic structures. The consistency across languages suggests that the underlying pronunciation model adapts well to different phonetic patterns, stress rules, and syllabic structures.
Testing across both high and medium-frequency word bands confirmed the model maintains accuracy regardless of word commonality, suggesting comprehensive phonetic coverage across varied vocabulary.
Testing Methodology
We developed our testing dataset through a structured, linguistics-based approach:
Reference Framework: We utilized the Leipzig Corpus as our foundation, which provides frequency analysis of words across multiple languages based on actual usage patterns.
Source Material: From this framework, we built a robust multilingual corpus containing 300,000 sentences collected from diverse news websites, ensuring broad topical and linguistic coverage.
Word Selection Criteria: Test words were carefully selected from two distinct frequency bands:
- High-frequency band: 2,500+ commonly used words that appear frequently in everyday language (examples: "find," "university," "about")
- Medium-frequency band: 2,000+ less common words that still appear regularly in written content (examples: "sneaking," "planners," "yells")
With this stratified approach, our pronunciation testing covered both essential vocabulary and more challenging terms that appear in standard usage.
Rather than evaluating isolated words, which can miss contextual pronunciation challenges, we placed each test word in a standardized carrier phrase. Each word appeared in a sentence following the pattern: "The word (first), is (second), it's not (third)."
This controlled context allows evaluation of how words are pronounced in neutral sentence environments, reflecting more realistic usage scenarios and consistent testing conditions.
All pronunciation assessments were conducted by native speakers of the respective languages being tested. This made sure that evaluations reflect authentic pronunciation standards for each language. Evaluators had no prior association with Murf to maintain objectivity in the assessment process.
We implemented a two-pass verification system:
- Each test word underwent independent review by two separate native-speaking annotators.
- Words were only marked as correctly pronounced when both reviewers independently agreed.
This consensus-based approach provides a higher standard of verification than single-reviewer methodologies, ensuring greater confidence in our accuracy measurements.
2nd Test - Challenging Terms Evaluation
Study Overview
While the 1st Test evaluated pronunciation accuracy across general vocabulary, the 2nd Test specifically focused on words with pronunciation difficulties. This test was designed to assess Murf’s capability to handle medical terms, proper nouns, and names that frequently cause difficulties for text-to-speech systems.
Results & Analysis
The evaluation shows Murf voices achieved greater than 95% pronunciation accuracy across all tested categories of challenging terms.

This performance indicates the system's robustness when handling specialized terminology that typically requires domain expertise for correct pronunciation.
Testing Methodology
We developed our testing dataset through a structured, linguistics-based approach:
- Medical Words: Selected from the NHS Health A-Z resource, these are specialized medical terms while excluding common health-related words like "heart" and "blood" that appear in everyday language.
- City Names: A diverse collection of global city names, which often include non-standard spellings, language-specific pronunciations, and regional variations.
- Last Names: Sourced from US Census data, representing a wide variety of ethnic origins and pronunciation patterns that frequently deviate from standard pronunciation rules.
These categories were selected based on two primary criteria:
- Their inherent pronunciation complexity like irregular spelling-to-sound mappings, specialized rules
- Their high frequency of use in practical applications like healthcare content, location-based services, personal communications
With this stratified approach, our pronunciation testing covered both essential vocabulary and more challenging terms that appear in standard usage.
The testing process involved:
- Collection of 8,000+ listening tests across the three categories
- Assessment using the same native-speaker verification methodology as the 1st Test
- Consistent application of evaluation criteria across all three challenging categories
3rd Test - Voice Quality & Naturalness
Study Overview
A competitive benchmarking study was conducted to evaluate Murf's text-to-speech technology against five leading competitors: AWS, Azure, ElevenLabs, Google, and OpenAI. The study covered four English variants (US, UK, Australian, and Indian) and eight additional languages.
The primary goal was to evaluate voice quality and naturalness through blind testing with native speakers. All voices were presented with conversational content for assessment.
Results & Analysis
The benchmarking results reveal that 8 out of 10 times Murf voices win on naturalness vs. competition. In this analysis, only definitive win or loss outcomes were considered, excluding 'Both are similar' responses.
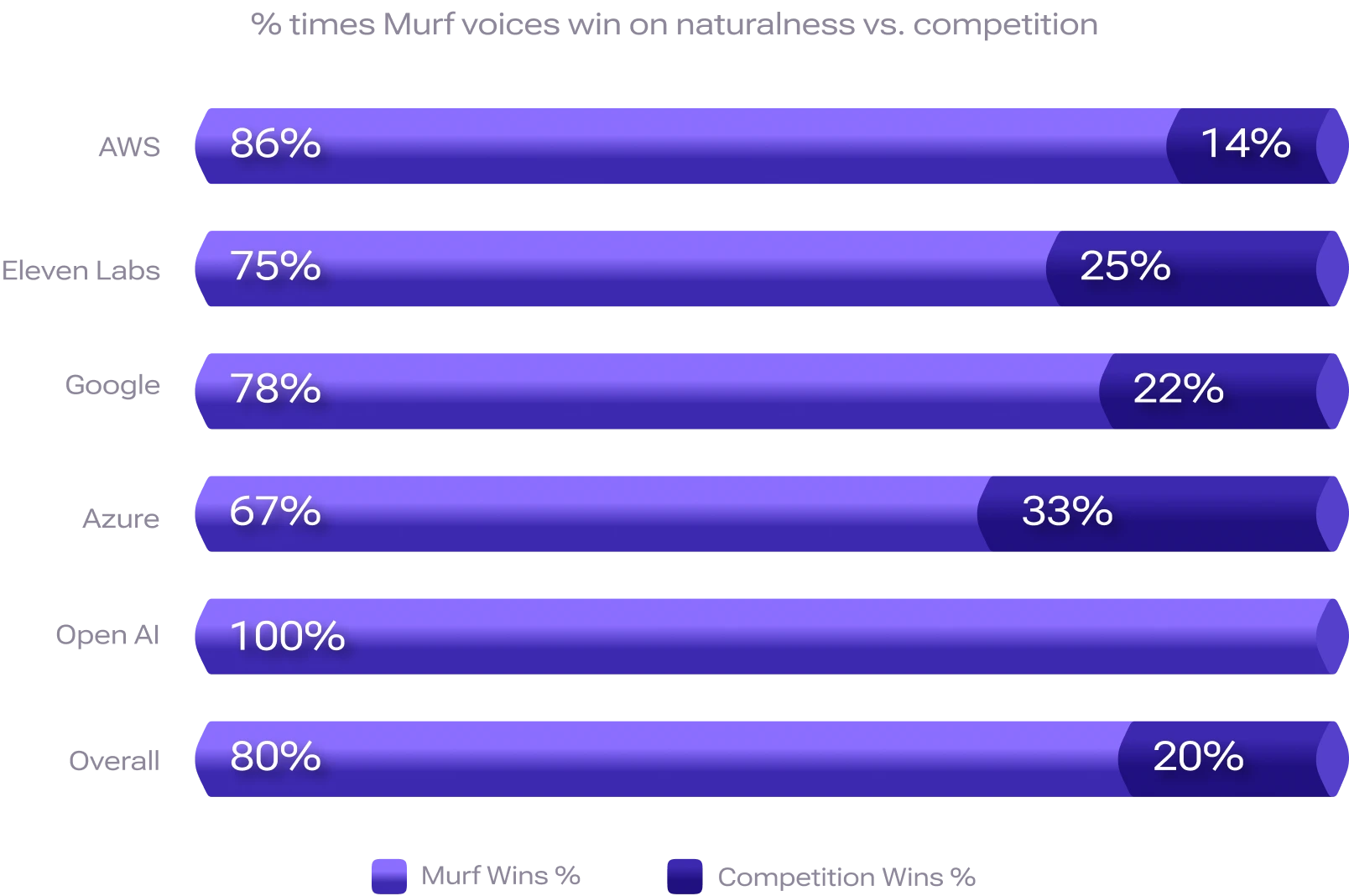
When broken down by competitors, Murf performed strongest against OpenAI (winning in 9 out of 9 languages tested), followed by AWS (winning in 6 out of 7 languages tested), Google (winning in 7 out of 9 languages tested), ElevenLabs (winning in 6 out of 8 languages tested), and Azure (winning in 6 out of 9 languages tested).
The number of languages varied by competitor based on what they supported at the time of evaluation.
Testing Methodology
All evaluators were native speakers of the respective language being tested, ensuring accurate assessment of pronunciation, accent, and naturalness. These participants were sourced through a third-party staffing agency and had no affiliation with Murf to maintain objectivity. All participants received compensation for their time, standardizing the evaluation process.
Participants used an evaluation tool designed for voice comparison. For each test, listeners were presented with two unlabeled voice samples. One from Murf and one from a competitor, without knowing which was which. Across the entire study, participants evaluated more than 11,000 audio sample pairs. They indicated their preference between Voice A, Voice B, or "Both are similar," and had the option to provide qualitative feedback explaining their choice. This combination of quantitative preference data and qualitative reasoning created a rich dataset for analysis.
Scripts were developed by language experts to replicate realistic scenarios and segmented into approximately 10-second clips for optimal evaluation. All scripts featured natural dialogue patterns typical of everyday conversations.
The third-party agency conducting the benchmarking visited each provider's website and filtered for the specific target accent or language. They selected the first available voice meeting the criteria and audio samples were generated directly via each provider's API with no post-processing or modifications.
Detailed Performance By Language
Win rates of 78% against Google, 71% against Azure, 68% against AWS, 60% against ElevenLabs, and 50% against OpenAI.

Win rates of 97% against OpenAI, 95% against AWS, 93% against Azure, 80% against ElevenLabs, and 63% against Google.
.webp)
Win rates of 96% against OpenAI,86% against Google, 74% against Azure, 72% against ElevenLabs, and 70% against AWS.
.webp)
Win rates of 100% against AWS, 98% against OpenAI, and 94% against Azure.
.webp)
Win rates of 94% against Eleven Labs, 89% against Open AI, 86% against Google, and 84% against AWS.
.webp)
Win rates of 84% against OpenAI, 76% against Azure, and 66% against ElevenLabs.
.webp)
Win rates of 97% against OpenAI, 95% against Azure, 88% against Google, and 56% against ElevenLabs.
.webp)
Win rates of 100% against Google, 98% against OpenAI, and 92% against AWS, but lower rates of 45% against Azure and 40% against ElevenLabs.
.webp)
Win rates of 96% against OpenAI, 46% against Google, and 35% against Azure.
.webp)
Voice Quality Factors and User Feedback
Analysis of participant comments revealed several key factors that influenced voice preferences:
Pronunciation accuracy emerged as a primary consideration, with listeners sensitive to correct handling of specialized terms, proper nouns, and abbreviations. The ability to deliver authentic accent-appropriate pronunciations also significantly impacted perceived quality.
Speech rhythm and pacing proved equally important to listener perception. Voices with appropriate pauses at commas, periods, and other punctuation were consistently preferred over those with unnatural breaks or rushed delivery. Listeners also valued natural speed variations that avoided monotony, with different expectations for different content types.
Emotional presence appropriate to the content type enhanced perceived quality, as did natural intonation variation with proper rises and falls in pitch. Proper handling of questions with appropriate rising intonation was specifically noted in several feedback comments.
Technical quality factors also played a role in evaluation. Listeners noted the absence of artifacts like glitches or robotic sounds, consistent volume throughout utterances (no fading out at sentence ends), and overall voice clarity as important quality indicators
Murf Voices Now Available on API
Murf voices are now available via REST API and Python SDK. Get started with 100,000 free characters and refer to our docs to get your first API call running in under 5 minutes. Easily generate high-quality speech across multiple languages and voices.