Eliminating the Lack of Diversity in Text to Speech
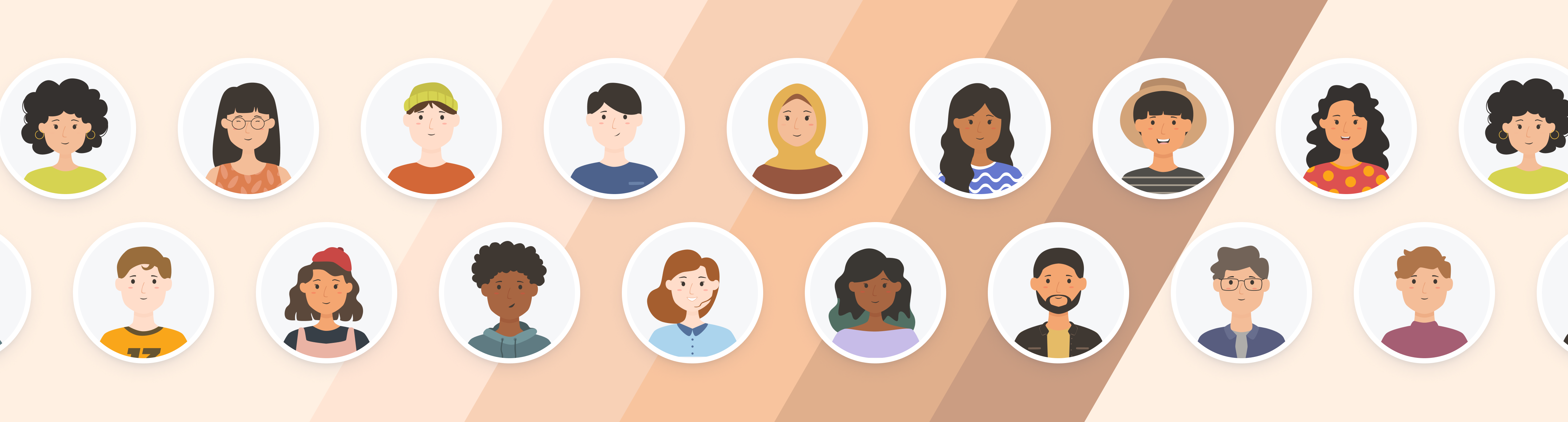
The science that goes into making machines talk like humans is very complex because our speech patterns are so nuanced. So, it’s not surprising that it has taken well over 200 years for AI voices to get from the first speaking machine which was able to simulate only a few recognizably human utterances to a Samuel L. Jackson voice clone delivering the weather report on Alexa today. Talking assistants like Siri, Google Assistant, and Alexa, now sound more human than before. Thanks to advances in AI, we’ve reached a point where it’s sometimes difficult to distinguish synthetic voices from real ones.
Despite such innovative developments, there still exist disparities in these text to speech (TTS) systems. Specifically, in how certain accents and words in particular languages are delivered. For example, voice AI mispronounces words in many Asian, African, and Latin languages. In fact, researchers at Stanford University recently published a study in which they reported findings from researching five automatic speech recognition systems. The average word error rate for white subjects was 19 percent compared to 35 percent for black subjects speaking in African-American Vernacular English.

Table of Contents
So, Why Does Such Bias Still Exist in TTS Systems?
While there are numerous possible reasons, a major cause boils down to the bias that prevails in the algorithms powering these TTS services. Many TTS systems are trained on limited sets of data, making them less effective. For example, the most commonly used dataset for TTS is, LibriSpeech, a corpus of approximately 1000 hours of 16kHz read English speech. The data for LibriSpeech is derived from read audiobooks from the LibriVox project and has been carefully segmented and aligned. Although LibriSpeech was started in 2015 and LibriVox in 2005, respectively, the majority of the books used for the audio recordings were written in the 19th century and before. This, in essence, means there certainly exists bias in terms of the lack of characters of color in these stories. Furthermore, the speakers that contributed to the LibriVox project recordings were not necessarily African Americans, Latinos, or Asians.
Moreover, LibriVox contains enough English content for a speech processing corpus, LibriSpeech, to be built from it but the same cannot be said for other languages. To state facts, LibriVox comprises over 60 thousand hours of content for English but comparatively less for other languages such as Greek, Latin, Japanese, and more. Another more recent dataset used by these voice AI systems, LibriTTS, addresses this issue.
LibriTTS is designed explicitly for text to speech. The new speech corpus is derived from the original audio and text materials of the LibriSpeech corpus and inherits the desired properties of the LibriSpeech corpus. LibriTTS corpus consists of 585 hours of speech data at a 24kHz sampling rate from 2,456 speakers and the corresponding texts. Despite the profusion of speech data through these datasets among others, it is nevertheless difficult to find datasets that allow training the models on prosodic and emotional aspects. This serves as a second major hindrance.
There must be a large amount of clean speech data from one speaker to train an end-to-end speech synthesizer from scratch which is not available for several low-resource languages. Besides, there is also an inadequacy of a reliable text normalization front-end and lexicon for these languages, which means phonemes are also not available for that particular text.
A third issue lies in the way the TTS systems are trained to recognize sound. At the phoneme level, these AI systems are tied to the phoneme set of the language a voice is built from. This is usually because modern speech synthesizers use recorded voice samples in a diphone database, allowing them to sound more natural. As a result, the phoneme set is restricted to the voice's language.
Correct prosody and pronunciation analysis from written text is also a major challenge today. Written text contains no explicit emotions and pronunciation of certain words is sometimes very anomalous. At a low-level synthesis, the discontinuities and contextual effects in wave concatenation methods are the most problematic.
Bridging the Digital Divide in Speech Synthesis AI
Eliminating bias means you have to be meticulous with the data these TTS algorithms use and take a more holistic approach to not just including more diverse data but also phonetic fundamentals. It is equally important to consider how the synthetic voices perform in different environments through focus groups. In other words, data must not only represent different dialects but genders as well, in order to reduce these race and gender biases and be more accurate.
Utilizing a technology that learns from multiple diverse data sets is a way of reducing the gaps and allowing all voices to be heard. It’s important to emphasize that the deep learning technology itself isn’t biased and the algorithm isn’t biased upfront but they can attain inherent biases if the data set provided does not accurately represent a population.
In order to limit as many of these biases as possible within AI, it’s crucial to make sure you are representing a wide range of people with various demographics. Bias-free AI models are important so that they work for every person and create meaningful results that help solve problems, not create them.
To put it simply, whether you’re building a TTS model from scratch, or fine-tuning an existing one, quality data should include a balanced representation of different voices across gender, age groups, race, and unique speakers. With the right mix of diverse data, your AI model will perform as envisaged: able to respond to the real world with all its variations.
Building Voice Tech that Reflects Diversity
The adoption and innovation of voice AI is gathering speed at an unprecedented pace. For AI technology to be truly useful to the world at large, however, it has to be globally representative. It’s time we recognize that we naturally 'build for our own' and make a conscious decision to test our voice innovations with a much broader group of people.
Aligning with the aspect of diversity in TTS, we at Murf have created a new range of male and female African American AI voices. Terrell is among the mature voices in Murf studio and one of the most impactful and inspirational voices in our newly launched collection of emotive AI voices. Whether you are looking for an impactful narration or creating awareness through a project, Terrell’s voice will fit right in. His voiceover style is inspirational, powerful, and works best for inspirational ads, powerful documentaries, and motivational podcasts. Want to leave an impression, use Terrell’s voice.
Marcus, on the other hand, is one of the most welcoming, firm, and authoritative voices. Marcus’ tone is casual, conversational, and fits well for e-learning, commercial, and explainer content.
In addition, we have included three new female African-American voices to our range of emotive TTS voices: Brianna, Michelle, and Naomi.
While Brianna's voice style adapts easily to scripts that are conversational or professional, Michelle is a more authoritative and mature voice that lends a formal tone to eLearning, corporate training, and employee onboarding content. Brianna's voice suits best for eLearning and explainer videos.
Naomi, on the other hand, is a more dynamic voice that has the power to inspire and convey empathy. Naomi's voice style works best for motivational advertisements, documentaries, and healthcare videos.
You can check out the new voices by logging into Murf Studio.
The diversity conversation has been slowly creeping into the world of AI voiceovers but the historically anonymous nature of the industry, together with a very strong bias toward a particular population of people, made it seem like a fruitless cause. There is still obviously so much that needs to be done, however, this is the first step!

FAQs
Why is accent diversity important in text to speech technology?
Accent diversity in text to speech is critical for cultural inclusivity and optimal user experience. Embracing various accents ensures users from diverse linguistic backgrounds can understand and comprehend the text to speech content. Moreover, accent diversity eliminates bias and fosters inclusion across global language spectrums. By representing the diverse language usage, TTS technology becomes accessible to a wider audience with custom voices and audio content.
How does text to speech technology address and incorporate various accents?
TTS technology incorporates a spectrum of accents via diverse training datasets. These datasets meticulously analyze and synthesize speech patterns across various linguistic groups, enabling accurate accent recognition and reproduction. The process entails extensive customization and employs accent recognition algorithms to guarantee the delivery of natural-sounding speech output. Through this approach, TTS technology ensures that users receive authentic and culturally inclusive auditory experiences, reflecting the diversity of language usage across different demographics.
In what ways can businesses benefit from incorporating diverse voices in text to speech applications?
Businesses benefit from diverse voices in TTS applications by enhancing accessibility and expanding their user base. By catering to diverse linguistic needs, businesses demonstrate cultural sensitivity and inclusivity, fostering enhanced user experience. Additionally, incorporating a diverse range of voices enables businesses to reach a broader audience and effectively communicate their message across different demographics. With a wide range of diverse voices, customization in text to speech can reach new heights by understanding the written text and producing natural-sounding voices to support students, businesses, and other use cases.
How important are diverse training datasets in text to speech technology?
Diverse training datasets in TTS technology are vital for eliminating bias and improving accent recognition accuracy. These datasets encompass a wide range of linguistic variations, including AI voices, accents, dialects, and speech patterns. By training on diverse datasets, TTS models learn to adapt to different linguistic nuances, enhancing their ability to produce natural-sounding speech across various accents. This helps increase the relevance of a platform to a wider demographic and empowers underrepresented communities through inclusivity.
How does text to speech technology recognize and reproduce different accents accurately?
TTS technology recognizes and reproduces different accents accurately through sophisticated algorithms and machine learning techniques. By analyzing phonetic variations and speech patterns specific to each accent in audio recordings, TTS models adapt their synthesis process to match the desired accent’s nuances for diverse audiences. Model refinement in text to speech is a continuous process as it also learns from the data inserted by the users.
How does text to speech technology enhance accessibility for users with diverse needs?
Text to speech technology enhances accessibility by providing alternative means of communication for users with diverse needs. It allows visually impaired individuals to access written content through auditory channels, promoting inclusivity and independence. Moreover, accessibility in text to speech enables users with learning disabilities or language barriers to comprehend and interact with digital content more effectively, breaking down communication barriers and fostering equal access to information.
Can users expect regular updates and improvements in text to speech models for better performance?
Yes, users can expect regular updates and improvements in text to speech models to enhance performance and address emerging needs. Continuous research and development efforts focus on refining TTS algorithms, expanding accent recognition capabilities, and improving speech synthesis quality. These updates ensure that TTS technology remains responsive to evolving user expectations and technological advancements, delivering optimal performance and user experiences.
How does the concept of inclusive technology apply to text to speech applications?
Inclusive technology in text to speech applications emphasizes the importance of accommodating diverse linguistic backgrounds and accessibility needs. By prioritizing cultural inclusivity, bias elimination, and user-centered design principles, TTS applications strive to create environments where all users feel represented and empowered. With the use of audio recordings or visual and auditory input, users with visual impairments can use the text to speech software for their needs. Inclusive technology fosters equal access to information and communication resources, promoting social equity and diversity in digital spaces.